In the equity market, a stock's valuation is often determined by comparing its market price to a fundamental anchor, such as the company's book value or earnings. Similarly, value investing in the credit market seeks to identify mispricings by determining whether a bond's credit spread adequately compensates for its risk.
A typical quantitative approach is to estimate the fair spread in a linear regression framework on credit spreads (Houweling and Van Zundert, 20171 ). The residual of the regression serves as a value measure, which is the difference between the estimated fair spread and the market spread. A large residual indicates that a bond is mispriced, while a small residual indicates that a bond is fairly priced.
Optimizing risk management with machine learning
However, linear regression models can’t handle more complex dependencies between risk measures (nonlinearities and interactions) easily, because the number of explanatory variables quickly becomes unmanageable. For example, five risk measures leads to ten interactions, but with ten risk measures, the number of interactions is already forty-five.
The idea is thus to use a model based on machine learning (ML), specifically so-called regression trees, to enhance the value factor. To better control for risk than traditional methods, regression trees can account for non-linearities and interaction effects, thereby reducing the bias toward riskier bonds and allowing investors to benefit more from true mispricings.
To see how successful the ML-based value factor is at reducing risk, we evaluate the exposures of the ML-based value factor to traditional corporate bond risks and compare it to a value factor with linear risk controls and a value factor with no controls.
Figure 1: Exposure to risk dimensions
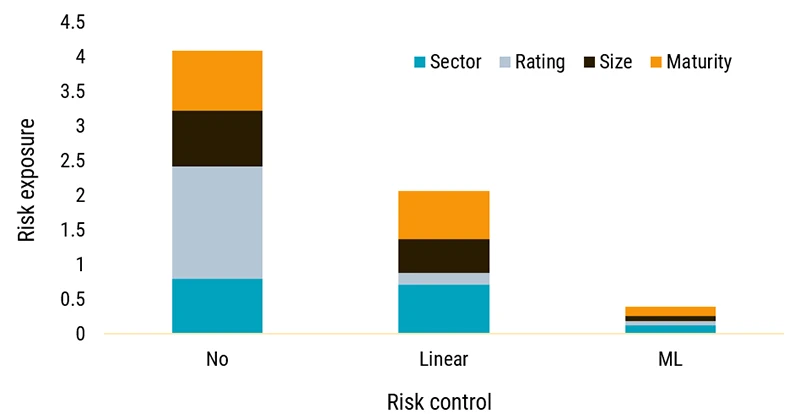
Source: Robeco, 2024.
Why choose ML to control risk?
For all value factors, we measure the exposure to credit ratings, sectors, issuer size groups, and maturity groups for each month and aggregate the active exposures over time. A lower risk exposure score indicates that a value factor is better at controlling a given risk dimension. The figure shows that using linear risk controls reduces the risk by half compared to having no controls at all, demonstrating the importance of controlling risk.
The figure also shows that using ML-based risk controls reduces risk even more. This significant reduction in risk makes the ML-based value factor less vulnerable to systematic shocks and therefore less risky, clearly demonstrating the advantages of an ML-based approach over traditional value factors in credit. This is one of the reasons why we also use an ML-based value factor in our Quant Credit products, such as Multi-Factor Credits and Multi-Factor High Yield.
Further information can be found either in our paper “True Value Investing in Credits through Machine Learning” or in an article on our website.
Footnote
1Houweling, Patrick and Jeroen van Zundert. 2017. “Factor Investing in the Corporate Bond Market.” Financial Analysts Journal 73 (2): 100-115.
Get the latest insights
Subscribe to our newsletter for investment updates and expert analysis.