In the past five years, the application of machine learning (ML) techniques for predicting stock returns has seen a significant surge. Numerous studies have confirmed that ML-based alpha models often outperform traditional, linear models in predicting cross-sectional equity returns.1 However, ML techniques are often referred to as “black boxes.” Something goes in, something comes out, but the inner workings of the algorithms remain obscure. This is where tools like Shapley values come into play – they help to understand why machine-learning models make certain predictions.2
For every prediction an ML model makes, Shapley values indicate the contribution of each variable (feature) to the prediction (target). Imagine we’re predicting future stock returns, and the model predicts an outperformance of 4% for a particular stock. Shapley values allow us to attribute this for instance as follows: 2% due to value, 1% due to momentum, and 1% due to quality.
Figure 1: Shapley plots for a boosted regression tree model predicting one-month-ahead returns.
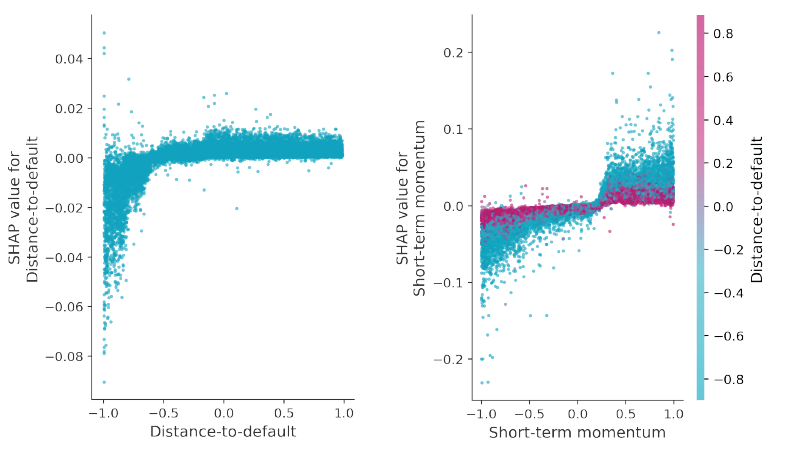
Source: Robeco, Refinitiv. The figure shows a Shapley scatter plot (left) and a Shapley dependence plot (right) for a boosted regression tree model predicting one-month ahead standardized returns. Shapley values are shown on the y-axis, with a Shapley value above 0 indicating that a feature has a positive impact on model predictions. The chart on the left shows the relation between distance-to-default and one-month-ahead returns. The chart on the right shows an interaction effect between short-term momentum (x-axis) and distance-to-default (color) for one-month-ahead returns. The boosted regression tree model is trained on one-month ahead relative returns. We include several dozen common as well as proprietary features whose ranks are cross-sectionally mapped into the [-1,1] interval. For missing values, the cross-sectional median is imputed. The model is trained on monthly data from January 1986 to December 2022, using all constituents of the MSCI World Index.
As technology advances, so do the opportunities for quantitative investors. By incorporating more data and leveraging advanced modelling techniques, we can develop deeper insights and enhance decision-making.
Furthermore, Shapley dependence plots can also illuminate the functional form between a feature and the target. Figure 1, for instance, illustrates potential nonlinearities and interaction effects in ML return prediction models. Shapley values are shown on the y-axis, with a Shapley value above 0 indicating that a feature has a positive impact on model predictions.
The chart on the left indicates a positive relationship between distance-to-default and expected returns. This pattern is consistent with the well-known low-risk effect, which suggests that higher risks are not necessarily rewarded with higher returns. However, this relationship is nonlinear: stocks closer to default exhibit a highly negative relation between distress risk and expected returns, while the relationship remains relatively flat for stocks far away from default.
Moreover, the chart on the right unveils an interaction effect between short-term momentum and distance-to-default.3 Generally, the Shapley plot indicates that stocks with high short-term momentum tend to have higher future returns. However, this effect is more pronounced for stocks with low distance-to-default (blue dots) than for stocks with high distance-to-default (red dots). This insight reveals that while stocks with low distance-to-default typically have lower expected returns, short-term momentum can discern between short-term winners and losers within this volatile group of stocks.
In conclusion, Shapley values play a pivotal role in transforming ML models from “black boxes” to “glass boxes.” The black boxes metaphor stems from the increased complexity of ML models and the difficulty in understanding the decision-making process behind predictions. Shapley values, however, quantify the contribution of each feature in the model to a specific prediction. They provide a transparent layer, allowing us to see and understand the impact and importance of individual variables on the predictions. This interpretability, akin to peering into a glass box, is paramount in assessing the trustworthiness of ML predictions and making informed investment decisions based on them.
Footnotes
1 See for instance, Gu, Kelly, and Xiu, 2020, “Empirical Asset Pricing via Machine Learning”, The Review of Financial Studies for the United States, Tobek and Hronec, 2021, “Does it pay to follow anomalies research? Machine learning approach with international evidence”, Journal of Financial Markets for developed markets, and Hanauer and Kalsbach, 2023, “Machine learning and the cross-section of emerging market stock returns”, Emerging Markets Review for emerging markets. For a discussion of the promises and pitfalls of ML, we also refer to and Leung, Lohre, Mischlich, Shea, and Stroh, 2021, “The Promises and Pitfalls of Machine Learning for Predicting Stock Returns”, The Journal of Financial Data Science, Blitz, Hoogteijling, and Lohre, 2023, “Researchers have just been scratching the surface of ML in asset management”, Robeco article, and Chen and Zhou, 2023, “Machine learning in finance: Why and how?”, Robeco article.
2 See Shapley, 1953. “A Value for n-person Games.” Contributions to the Theory of Games. Annals of Mathematical Studies.
3 Short-term momentum is a proprietary signal with a lookback of one month that captures systematic short-term momentum effects such as industry, country, and factor momentum.