The study by David Blitz, Matthias Hanauer, Tobias Hoogteijling and Clint Howard on ‘The term structure of machine learning alpha’ emphasizes the importance of design choices in the effectiveness of ML models in real-world applications. Previously, the focus of the majority of studies and strategies was centered around predicting stock returns over one-month horizons. Although these short-horizon strategies exhibited impressive gross alphas, they were mainly profitable before 2004. Their performance deteriorated significantly after 2004 due to high transaction costs resulting from their higher turnover.
In contrast, our research shows that ML models trained on longer prediction horizons (e.g., three, six, or twelve months) performed considerably better in generating net positive returns, especially in the more recent period from 2004 to 2021. The longer-horizon strategies required less frequent trading, thereby drastically reducing transaction costs. These strategies also loaded more on slower signals, such as quality and value, which proved to be more resilient and profitable in the long term.
Furthermore, applying efficient portfolio construction rules – akin to a buy-hold cost mitigation approach – proved to be beneficial across all prediction horizons. This technique significantly reduced turnover, thus compensating for the reduced raw portfolio returns.
Our study’s findings have critical implications for both academia and investment practitioners. For researchers, the paper highlights the need to broaden the focus beyond one-month return predictions and to understand the behavior of ML models across various prediction horizons. For investment practitioners, the findings advocate for aligning ML model training with the intended application and portfolio holding period. By doing so, investors can capitalize on the reduced turnover and improved net performance offered by strategies based on longer prediction horizons.
Figure1: Hypothetical cumulative performance of different training horizons over future months
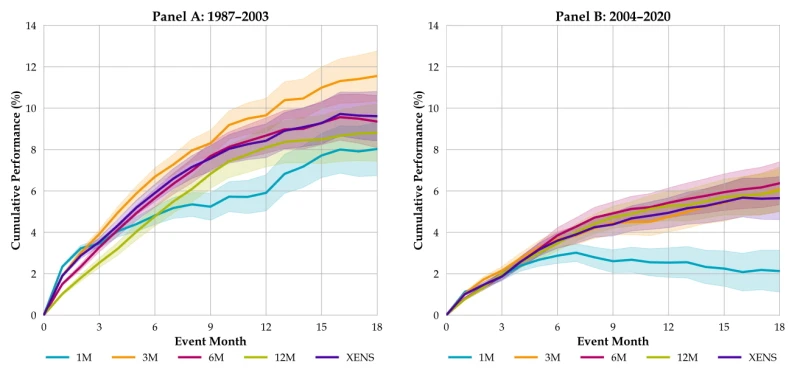
Source: CRSP, Open Source Asset Pricing, Robeco. Note: This figure plots the hypothetical cumulative average performance of the top-minus-bottom value-weighted decile portfolios for an ensemble of machine learning models trained on different prediction horizons, when holding the portfolios without rebalancing.
To summarize, although ML models focused on short-term predictions have lost their edge in recent years, we believe that by adopting longer prediction horizons and efficient portfolio construction rules there is potential to unlock significant net returns. These findings underscore the importance of design choices and call for more extensive research on various prediction horizons to optimize investment strategies using ML models.
Get the latest insights
Subscribe to our newsletter for investment updates and expert analysis.