Traditional risk indicators like stock beta and return volatility are useful but limited as they rely on historical data. To better estimate tail risk, Robeco has incorporated advanced measures such as distance-to-default (DtD) since 2011, and more recently in 2021, a machine learning (ML) risk signal that identifies complex market patterns. This note reviews their performance, particularly focusing on out-of-sample results. It demonstrates that DtD and ML signals have outperformed traditional measures in real-life investment strategies, supported by practical examples and analysis.
What are the DtD and ML signals?
The distance-to-default (DtD) signal, inspired by the Merton model1 , captures how close a firm is to defaulting on its debt. It’s a key concept in credit risk modelling, used by analysts and bond investors to assess a company's financial stability and the likelihood of credit default. In this model, stock equity is seen as a call option on the company's total value, including liabilities. This value is influenced by the volatility of the company's asset market value. The forward-looking nature of DtD offers additional insights compared to traditional metrics. Since 2011, an enhanced version of this proprietary distress risk measure is a negative screening tool for all our Quantitative Equity strategies, and has been integral to our stock selection model for Conservative Equities.2
The machine learning risk signal, introduced to our Quantitative Equity models in 2021, is trained to identify firms likely to suffer severe stock price crashes. ML techniques have several advantages, such as adapting to data patterns and capturing complex relationships like nonlinearities and interaction effects.3 For example, the empirical relationship between a firm’s financial leverage and its risk level is not linear. While firms with low or average leverage tend to have an average distress risk, those with high leverage are much more likely to experience significant price crashes. ML techniques excel at identifying such nonlinear relationships. 4
Active Quant: finding alpha with confidence
Blending data-driven insights, risk control and quant expertise to pursue reliable returns.
Data and analysis
Our evaluation focuses on comparing the out-of-sample performance of three distress indicators since their incorporation into real-life investment strategies:
50/50 combination of beta and volatility
DtD signal
ML distress signal
For this we include a year-by-year analysis, examples of individual stock price crashes, power curves and portfolio sorts.
Year-by-year analysis: Since integrating DtD into Robeco's strategies in 2011, the annual performance of the riskiest stocks has been tracked. In strong market years like 2013 and 2019, these stocks sometimes outperformed the market. However, during downturns (e.g., 2018 and 2022), as expected the high DtD stocks suffered more significant losses.Notably, during negative market years, emerging markets experienced more substantial drawdowns than developed markets. On average, DtD outperformed traditional beta/volatility measures by about 2 to 3% annually in both developed as well as emerging markets.
Individual stock examples: In 2022, many companies experienced substantial drops in market value, with the top ten losers declining by an average of 69.8%. Noteworthy cases include Silicon Valley Bank and Snap, which had poor DtD and ML risk signal scores at the beginning of the year. This highlights how DtD and ML can effectively predict individual stock crashes complementing traditional risk factors.
Power curves: A statistical tool commonly used to assess prediction accuracy is the power curve. In this analysis, all three indicators (beta & volatility, DtD, and ML) outperformed the no-predictability benchmark. The power curves for DtD and ML were further from the 45-degree line than the beta & volatility curve, indicating their superior predictive power. The ML signal had the highest area under the curve (AUC) score, followed by DtD and then the beta-volatility combination.
Figure 1 | Power curves for various predictors of distress
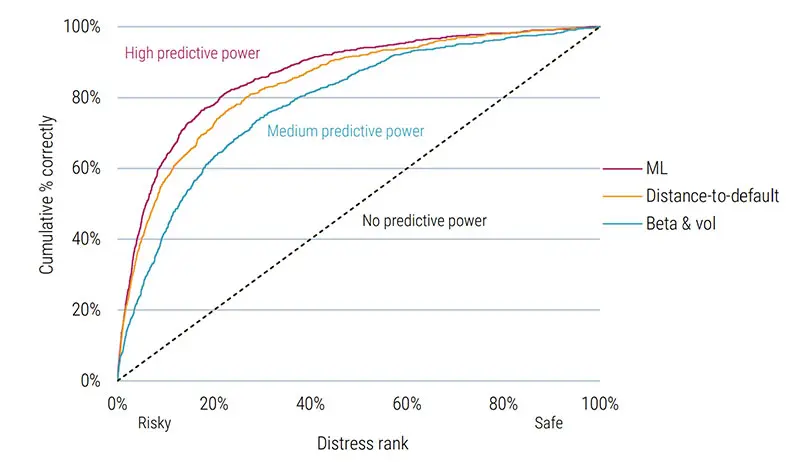
Global Developed Markets 2002-2023. Source: Robeco, MSCI, DataStream, Compustat and Worldscope, 2023.
Portfolio sorts: The analysis examined the 10% of stocks with the highest risk scores (beta & volatility, DtD, and ML). Stocks in these high-risk portfolios typically underperformed the market. The underperformance was more pronounced for DtD and ML compared to the beta-volatility basket. This indicates that advanced measures like DtD and ML are more effective in detecting risky stocks, and avoiding or shorting these stocks has historically led to higher returns.
Conclusion
This article presents detailed evaluations of proprietary tail risk predictors used in all of our Quantitative Equity strategies in general and Conservative Equities in particular, focusing on the comparison between traditional and advanced measures. Our analysis, which includes a year-by-year review, individual stock examinations, power curves and portfolio sorts, indicates that DtD and ML risk signals have added a distinct dimension to risk prediction since their inclusion in 2011 and 2021 respectively. These findings suggest a potential for improved identification of distressed stocks, which is a key consideration in strategy development and risk management.
Footnotes
1 Merton, R. C. (1974). On the pricing of corporate debt: The risk structure of interest rates. The Journal of finance, 29(2), 449-470.
2 How distress risk improves low volatility strategies: lessons learned since 2006, Joop Huij, Pim van Vliet, Weili Zhou and Wilma de Groot, Robeco Research Paper, February 2012
3 For an overview of the use of Machine Learning for asset management we refer to: Blitz, D., Hoogteijling, T., Lohre, H., & Messow, P. (2023). How can machine learning advance quantitative asset management? The Journal of Portfolio Management, 49(9), 78-95.
4 A more extensive description of the Machine Learning approach was published in 2021, at the time of introduction in our Quantitative Models, and can be found at: Forecasting stock crash risk with machine learning.
Discover the value of quant
Subscribe for cutting-edge quant strategies and insights.