Choices choices
The paper by Minghui Chen, Matthias Hanauer, and Tobias Kalsbach, titled ‘Design choices, machine learning, and the cross-section of stock returns’, identifies several key design choices researchers have to make when training ML models. For instance, when setting the prediction (target) variable, should the researcher employ the excess return over the risk-free rate or the abnormal return relative to the market? Is it better to use a continuous target variable or are categories, such as outperformers vs. underperformers, preferable? Is it better to train models based on a rolling window that leads to more adaptive models, or are models based on expanding windows superior, thanks to the availability of more training data?
To assess the importance of such choices, the authors identify seven such key design choices and examine all the ensuing possible combinations, resulting in a total of 1,056 ML models. In this way, the study trains each model on a common set of signals (features) for the US stock market and evaluates their out-of-sample performance using hypothetical top-minus-bottom decile portfolios.
Figure 1 reveals that portfolio returns vary substantially across different model designs, with monthly mean returns ranging from 0.13% to 1.98% and annualized Sharpe ratios ranging from 0.08 to 1.82.1 This variation highlights the substantial impact of human design choices on the performance of ML strategies.
Figure 1 | Cumulative performance of machine learning strategies
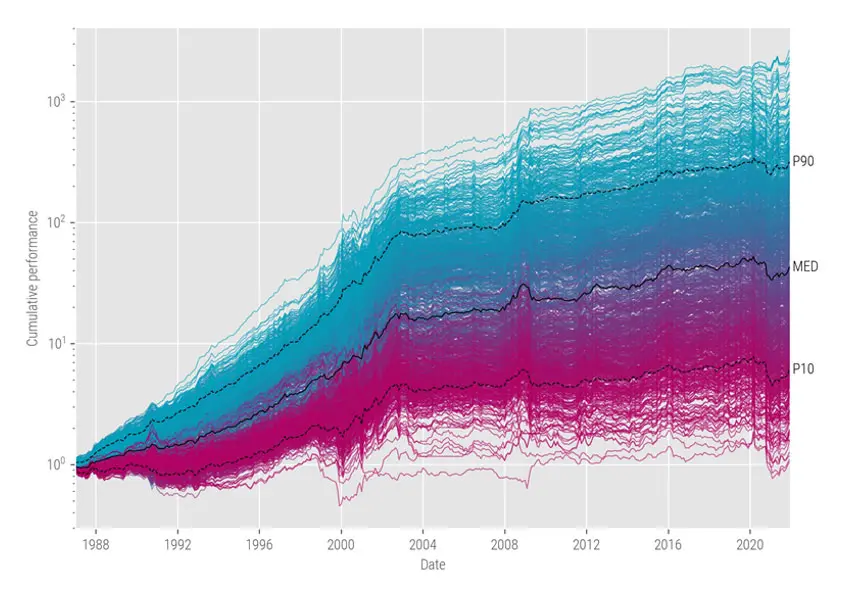
Source: Robeco, Chen et al. (2024). This figure shows the cumulative performance of a USD 1 initial investment in long-short ML portfolios for each possible combination of the research design choices. For each ML model and month, we first cross-sectionally sort all stocks based on their one-month-ahead return predictions. We then construct the value-weighted long-short portfolios by going long the top decile and short the bottom decile stocks. The solid black line represents the strategy with the median cumulative performance for each month, and the dashed black lines represent the 10th and 90th percentiles of each month, respectively. The sample period is from January 1987 to December 2021.
Machine learning models: Separating the wheat from the chaff
Having documented the substantial variation in the performance of ML models, the study also provides actionable guidance for ML model design:
Ensembles of ML models typically outperform individual algorithms.
The choice of target variable depends on the investment objective:
o For identifying relative winners and losers among stocks, predicting stock returns over the market rather than the risk-free rate is better.
o If the goal is to achieve high market-risk-adjusted returns, CAPM beta-adjusted returns are better.Non-linear ML models are more likely to outperform their linear counterparts when:
o using abnormal returns relative to the market as the target variable,
o employing continuous target returns, or
o adopting expanding training windows.
Conclusion
While computational infrastructure, ML algorithms, and data have become significantly more accessible over the past decade or two, model design remains a critical component of success. At first glance, it might seem that an ML investment strategy only requires a few basic elements: cloud computing space, generic factor data, some Python packages, and a couple of data scientists. However, this approach often lacks the crucial domain knowledge that Robeco has cultivated over 20 years in quant investing. That’s why in financial markets, where the signal-to-noise ratio is low and the risk of overfitting high, investment experience, and economic intuition still play a pivotal role. Robeco’s extensive expertise ensures that ML models focus on meaningful patterns and avoid common pitfalls, bridging the gap between technology and investment insight.
Read the full paperFootnote
1Please note that these are hypothetical gross returns for long-minus-short strategies that do not consider any transaction costs. We investigated the impact of transaction costs on ML strategies in our study ‘The term structure of machine learning alpha’.
Discover the value of quant
Subscribe for cutting-edge quant strategies and insights.