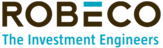
Disclaimer Robeco Switzerland Ltd.
The information contained on these pages is solely for marketing purposes.
Access to the funds is restricted to (i) Qualified Investors within the meaning of art. 10 para. 3 et sequ. of the Swiss Federal Act on Collective Investment Schemes (“CISA”), (ii) Institutional Investors within the meaning of art. 4 para. 3 and 4 of the Financial Services Act (“FinSA”) domiciled Switzerland and (iii) Professional Clients in accordance with Annex II of the Markets in Financial Instruments Directive II (“MiFID II”) domiciled in the European Union und European Economic Area with a license to distribute / promote financial instruments in such capacity or herewith requesting respective information on products and services in their capacity as Professional Clients.
The Funds are domiciled in Luxembourg and The Netherlands. ACOLIN Fund Services AG, postal address: Leutschenbachstrasse 50, CH-8050 Zürich, acts as the Swiss representative of the Fund(s). UBS Switzerland AG, Bahnhofstrasse 45, 8001 Zurich, postal address: Europastrasse 2, P.O. Box, CH-8152 Opfikon, acts as the Swiss paying agent.
The prospectus, the Key Investor Information Documents (KIIDs), the articles of association, the annual and semi-annual reports of the Fund(s) may be obtained, on simple request and free of charge, at the office of the Swiss representative ACOLIN Fund Services AG. The prospectuses are also available via the website https://www.robeco.com/ch.
Some funds about which information is shown on these pages may fall outside the scope of CISA and therefore do not (need to) have a license from or registration with the Swiss Financial Market Supervisory Authority (FINMA).
Some funds about which information is shown on this website may not be available in your domicile country. Please check the registration status in your respective domicile country. To view the Robeco Switzerland Ltd. products that are registered/available in your country, please go to the respective Fund Selector, which can be found on this website and select your country of domicile.
Neither information nor any opinion expressed on this website constitutes a solicitation, an offer or a recommendation to buy, sell or dispose of any investment, to engage in any other transaction or to provide any investment advice or service. An investment in a Robeco Switzerland Ltd. product should only be made after reading the related legal documents such as prospectuses, annual and semi-annual reports.
By clicking “I agree” you confirm that you/the company you represent falls under one of the above-mentioned categories of addressees and that you have read, understood and accept the terms of use for this website.
Quantitative investing
LASSO regression
LASSO is an acronym that stands for ‘least absolute shrinkage and selection operator’. It is associated with a machine learning technique – LASSO regression – that performs both shrinkage and variable selection to simplify linear regression models and prevent overfitting.
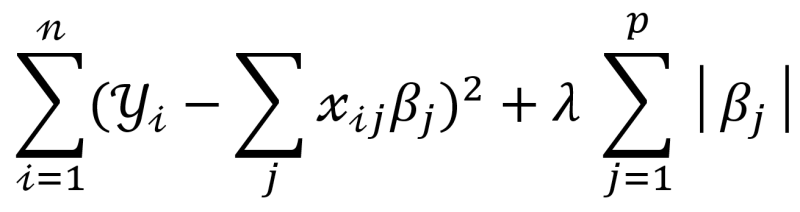
Where
λ is amount of shrinkage or penalty
λ = 0 implies all features are considered as no parameters are eliminated
λ = ∞ implies no feature is considered
A linear regression allows you to determine if there is a relationship between variables. For example, it can quantify the relationship between a dependent variable (crop yields) and explanatory variables (soil fertility,temperature, water quality, etc.). But in cases where there are many candidate variables to explain crop yields, the statistical model can become complex and difficult to process.
The LASSO regression is helpful in such instances as it can select variables based on their importance. This is achieved through a process called shrinkage, a method which imposes a penalty to reduce the absolute size of the regression coefficients. Although reduced in magnitude, the most important variables will continue to reflect material coefficients, while the less-contributing variables will exhibit values close to zero or even zero.
Through this process, it identifies which variables to keep and which ones to exclude, based on the size of their coefficients. Using our example, the technique would gradually select the variables which best predict crop yields, beginning with the most important one before working its way through the list. At some point, adding more variables would no longer improve the prediction accuracy of the model sufficiently, but instead it would add substantial complexity.
Therefore, the technique allows you to simplify a model by reducing the number of parameters in a regression and precluding potential data noise. It also enables you to guard against overfitting by eliminating variables with little explanatory power, potentially making the model more robust across different datasets. Additionally, it can help optimize models with high multicollinearity as it can choose between correlated explanatory variables.
In general, the LASSO regression is a basic machine learning (ML) technique that can be used for many applications. It is essentially a standard linear regression with a slight twist. Contrary to more sophisticated ML techniques, however, it is not able to pick up non-linear relationships between variables.
For our quant investing platform, it has the potential to help fine-tune models by assisting us with variable selection. For instance, we have used it to select company characteristics that have linear predictive value for risk and returns. We have also used it to identify which industries lead or lag others in terms of returns.
See also
As technology advances, so do the opportunities for quantitative investors. By incorporating more data and leveraging advanced modelling techniques, we can develop deeper insights and enhance decision-making.