In the past five years, the application of machine learning (ML) techniques for predicting stock returns has seen a significant surge. Numerous studies have confirmed that ML-based alpha models often outperform traditional, linear models in predicting cross-sectional equity returns.1 However, ML techniques are often referred to as “black boxes.” Something goes in, something comes out, but the inner workings of the algorithms remain obscure. This is where tools like Shapley values come into play – they help to understand why machine-learning models make certain predictions.2
For every prediction an ML model makes, Shapley values indicate the contribution of each variable (feature) to the prediction (target). Imagine we’re predicting future stock returns, and the model predicts an outperformance of 4% for a particular stock. Shapley values allow us to attribute this for instance as follows: 2% due to value, 1% due to momentum, and 1% due to quality.
Figure 1: Shapley plots for a boosted regression tree model predicting one-month-ahead returns.
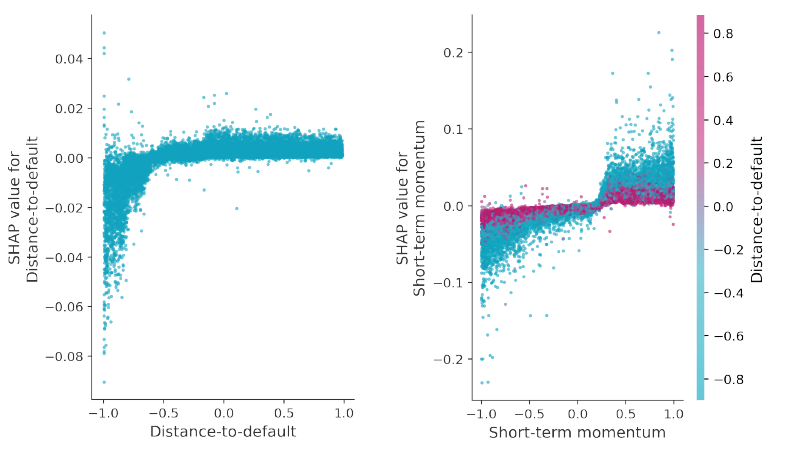
Source: Robeco, Refinitiv. The figure shows a Shapley scatter plot (left) and a Shapley dependence plot (right) for a boosted regression tree model predicting one-month ahead standardized returns. Shapley values are shown on the y-axis, with a Shapley value above 0 indicating that a feature has a positive impact on model predictions. The chart on the left shows the relation between distance-to-default and one-month-ahead returns. The chart on the right shows an interaction effect between short-term momentum (x-axis) and distance-to-default (color) for one-month-ahead returns. The boosted regression tree model is trained on one-month ahead relative returns. We include several dozen common as well as proprietary features whose ranks are cross-sectionally mapped into the [-1,1] interval. For missing values, the cross-sectional median is imputed. The model is trained on monthly data from January 1986 to December 2022, using all constituents of the MSCI World Index.
Furthermore, Shapley dependence plots can also illuminate the functional form between a feature and the target. Figure 1, for instance, illustrates potential nonlinearities and interaction effects in ML return prediction models. Shapley values are shown on the y-axis, with a Shapley value above 0 indicating that a feature has a positive impact on model predictions.
The chart on the left indicates a positive relationship between distance-to-default and expected returns. This pattern is consistent with the well-known low-risk effect, which suggests that higher risks are not necessarily rewarded with higher returns. However, this relationship is nonlinear: stocks closer to default exhibit a highly negative relation between distress risk and expected returns, while the relationship remains relatively flat for stocks far away from default.
Moreover, the chart on the right unveils an interaction effect between short-term momentum and distance-to-default.3 Generally, the Shapley plot indicates that stocks with high short-term momentum tend to have higher future returns. However, this effect is more pronounced for stocks with low distance-to-default (blue dots) than for stocks with high distance-to-default (red dots). This insight reveals that while stocks with low distance-to-default typically have lower expected returns, short-term momentum can discern between short-term winners and losers within this volatile group of stocks.
In conclusion, Shapley values play a pivotal role in transforming ML models from “black boxes” to “glass boxes.” The black boxes metaphor stems from the increased complexity of ML models and the difficulty in understanding the decision-making process behind predictions. Shapley values, however, quantify the contribution of each feature in the model to a specific prediction. They provide a transparent layer, allowing us to see and understand the impact and importance of individual variables on the predictions. This interpretability, akin to peering into a glass box, is paramount in assessing the trustworthiness of ML predictions and making informed investment decisions based on them.
Footnotes
1 See for instance, Gu, Kelly, and Xiu, 2020, “Empirical Asset Pricing via Machine Learning”, The Review of Financial Studies for the United States, Tobek and Hronec, 2021, “Does it pay to follow anomalies research? Machine learning approach with international evidence”, Journal of Financial Markets for developed markets, and Hanauer and Kalsbach, 2023, “Machine learning and the cross-section of emerging market stock returns”, Emerging Markets Review for emerging markets. For a discussion of the promises and pitfalls of ML, we also refer to and Leung, Lohre, Mischlich, Shea, and Stroh, 2021, “The Promises and Pitfalls of Machine Learning for Predicting Stock Returns”, The Journal of Financial Data Science, Blitz, Hoogteijling, and Lohre, 2023, “Researchers have just been scratching the surface of ML in asset management”, Robeco article, and Chen and Zhou, 2023, “Machine learning in finance: Why and how?”, Robeco article.
2 See Shapley, 1953. “A Value for n-person Games.” Contributions to the Theory of Games. Annals of Mathematical Studies.
3 Short-term momentum is a proprietary signal with a lookback of one month that captures systematic short-term momentum effects such as industry, country, and factor momentum.
免責聲明
本文由荷宝海外投资基金管理(上海)有限公司(“荷宝上海”)编制, 本文内容仅供参考, 并不构成荷宝上海对任何人的购买或出售任何产品的建议、专业意见、要约、招揽或邀请。本文不应被视为对购买或出售任何投资产品的推荐或采用任何投资策略的建议。本文中的任何内容不得被视为有关法律、税务或投资方面的咨询, 也不表示任何投资或策略适合您的个人情况, 或以其他方式构成对您个人的推荐。 本文中所包含的信息和/或分析系根据荷宝上海所认为的可信渠道而获得的信息准备而成。荷宝上海不就其准确性、正确性、实用性或完整性作出任何陈述, 也不对因使用本文中的信息和/或分析而造成的损失承担任何责任。荷宝上海或其他任何关联机构及其董事、高级管理人员、员工均不对任何人因其依据本文所含信息而造成的任何直接或间接的损失或损害或任何其他后果承担责任或义务。 本文包含一些有关于未来业务、目标、管理纪律或其他方面的前瞻性陈述与预测, 这些陈述含有假设、风险和不确定性, 且是建立在截止到本文编写之日已有的信息之上。基于此, 我们不能保证这些前瞻性情况都会发生, 实际情况可能会与本文中的陈述具有一定的差别。我们不能保证本文中的统计信息在任何特定条件下都是准确、适当和完整的, 亦不能保证这些统计信息以及据以得出这些信息的假设能够反映荷宝上海可能遇到的市场条件或未来表现。本文中的信息是基于当前的市场情况, 这很有可能因随后的市场事件或其他原因而发生变化, 本文内容可能因此未反映最新情况,荷宝上海不负责更新本文, 或对本文中不准确或遗漏之信息进行纠正。