In the equity market, a stock's valuation is often determined by comparing its market price to a fundamental anchor, such as the company's book value or earnings. Similarly, value investing in the credit market seeks to identify mispricings by determining whether a bond's credit spread adequately compensates for its risk.
A typical quantitative approach is to estimate the fair spread in a linear regression framework on credit spreads (Houweling and Van Zundert, 20171 ). The residual of the regression serves as a value measure, which is the difference between the estimated fair spread and the market spread. A large residual indicates that a bond is mispriced, while a small residual indicates that a bond is fairly priced.
Active Quant: finding alpha with confidence
Blending data-driven insights, risk control and quant expertise to pursue reliable returns.
Optimizing risk management with machine learning
However, linear regression models can’t handle more complex dependencies between risk measures (nonlinearities and interactions) easily, because the number of explanatory variables quickly becomes unmanageable. For example, five risk measures leads to ten interactions, but with ten risk measures, the number of interactions is already forty-five.
The idea is thus to use a model based on machine learning (ML), specifically so-called regression trees, to enhance the value factor. To better control for risk than traditional methods, regression trees can account for non-linearities and interaction effects, thereby reducing the bias toward riskier bonds and allowing investors to benefit more from true mispricings.
To see how successful the ML-based value factor is at reducing risk, we evaluate the exposures of the ML-based value factor to traditional corporate bond risks and compare it to a value factor with linear risk controls and a value factor with no controls.
Figure 1: Exposure to risk dimensions
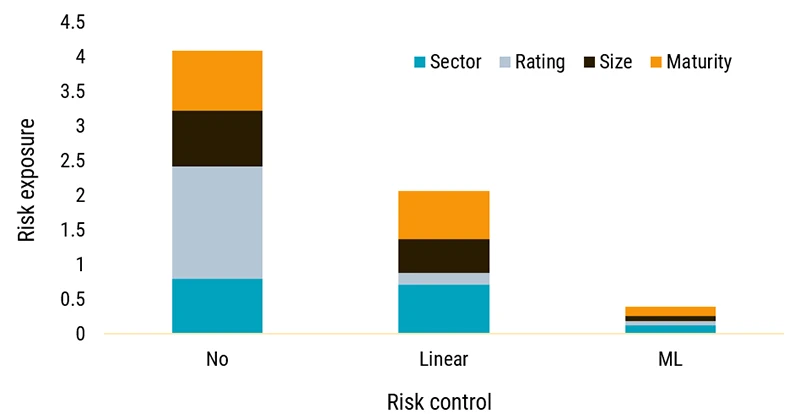
Source: Robeco, 2024.
Why choose ML to control risk?
For all value factors, we measure the exposure to credit ratings, sectors, issuer size groups, and maturity groups for each month and aggregate the active exposures over time. A lower risk exposure score indicates that a value factor is better at controlling a given risk dimension. The figure shows that using linear risk controls reduces the risk by half compared to having no controls at all, demonstrating the importance of controlling risk.
The figure also shows that using ML-based risk controls reduces risk even more. This significant reduction in risk makes the ML-based value factor less vulnerable to systematic shocks and therefore less risky, clearly demonstrating the advantages of an ML-based approach over traditional value factors in credit. This is one of the reasons why we also use an ML-based value factor in our Quant Credit products, such as Multi-Factor Credits and Multi-Factor High Yield.
Further information can be found either in our paper “True Value Investing in Credits through Machine Learning” or in an article on our website.
Footnote
1Houweling, Patrick and Jeroen van Zundert. 2017. “Factor Investing in the Corporate Bond Market.” Financial Analysts Journal 73 (2): 100-115.
獲取最新市場觀點
訂閱我們的電子報,時刻把握投資資訊和專家分析。
Important information
The contents of this document have not been reviewed by the Securities and Futures Commission ("SFC") in Hong Kong. If you are in any doubt about any of the contents of this document, you should obtain independent professional advice. This document has been distributed by Robeco Hong Kong Limited (‘Robeco’). Robeco is regulated by the SFC in Hong Kong. This document has been prepared on a confidential basis solely for the recipient and is for information purposes only. Any reproduction or distribution of this documentation, in whole or in part, or the disclosure of its contents, without the prior written consent of Robeco, is prohibited. By accepting this documentation, the recipient agrees to the foregoing This document is intended to provide the reader with information on Robeco’s specific capabilities, but does not constitute a recommendation to buy or sell certain securities or investment products. Investment decisions should only be based on the relevant prospectus and on thorough financial, fiscal and legal advice. Please refer to the relevant offering documents for details including the risk factors before making any investment decisions. The contents of this document are based upon sources of information believed to be reliable. This document is not intended for distribution to or use by any person or entity in any jurisdiction or country where such distribution or use would be contrary to local law or regulation. Investment Involves risks. Historical returns are provided for illustrative purposes only and do not necessarily reflect Robeco’s expectations for the future. The value of your investments may fluctuate. Past performance is no indication of current or future performance.