Investors show increasing interest in thematic investing, with active funds managed out of Europe having received about EUR 160 billion of new investor money in the last five years.1 Thematic investing focuses on long-term secular themes and the companies actively involved in and benefiting from them. Examples of such secular themes include microcomputers (PC), the internet, smartphones and connected devices, athleisure wear, and social media – all of which have unfolded over the past several decades. Meanwhile, current-day secular themes may include vehicle electrification, artificial intelligence (AI), and clean energy transition.
Thematic investing is typically carried out by fundamental investors, using human intuition and personal judgments. Strategies cover a wide range of structural changes: technological, regulatory and socioeconomic, and thematic investors count on their analytical edge and expectation biases from other investors to anticipate and take advantage of those structural changes.
Quantitative augmentations
As established and successful as this approach is, we believe quantitative methods can improve the thematic investment process in two main ways. Firstly, in the development of thematic hypotheses. The challenge of identifying these is that humans have limited mental, physical, and attention bandwidth. But this issue doesn’t apply to quantitative algorithms because machines can process vast amounts of information quickly, and they don’t get tired. With the appropriate algorithm and input, machines can identify emerging and trending themes across all companies in a strategy’s investment universe, picking up themes that human investors might have missed or would pick up at a much later time.
Secondly, algorithms do not become emotionally attached to an investment theme or any particular stocks associated with a given theme. Humans can lose objectivity due to the amount of time involved in picking out investments and the emotional attachment formed with their investments. Conversely algorithms evaluate investments based purely on how they are instructed. This may allow investors to exit themes at an appropriate stage as well, when the data no longer justifies such investments.
Quantitative techniques applied
Several components are necessary to implement a quantitative approach to theme investing. As outlined in Figure 1, there are three main techniques.
Figure 1: Techniques used in quantitative theme investing
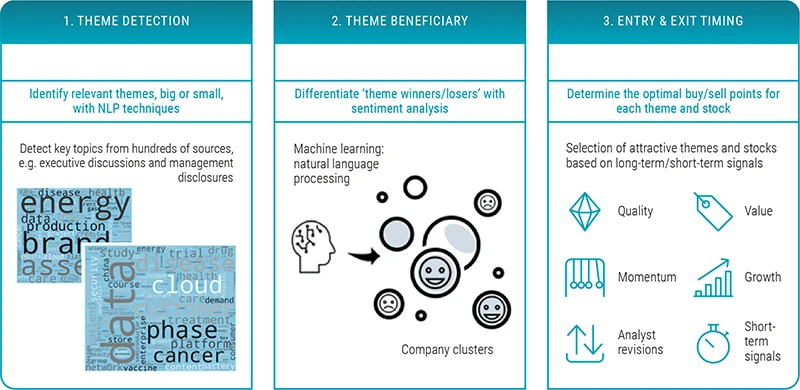
Source: Robeco, 2024.
We use natural language processing (NLP) to carry out theme detection, the first technique. For example, texts from various sources may be written about areas related to biomedical engineering, from research and product development to expected patient benefits to revenue streams and market growth. If these texts are relatively infrequent, the topic detection algorithm will not identify them as a theme. However, if these texts are frequent, then NLP will flag biomedical engineering as a theme. With the same algorithm, not only will the themes themselves be detected, but also companies associated with the themes.
In this example, emergence of advanced biomedical engineering may benefit companies that work on those advanced treatments, but for more traditional therapeutic companies, this may not be a welcome development. So for the second step, we apply another NLP tool called sentiment analysis to identify the sentiment of each company associated with the identified theme. This way, we can identify theme beneficiaries or winners (positive sentiment) and theme detractors or losers (negative sentiment).
A theme winner might be a great long-term investment, but over the shorter-term horizon, it might be overvalued and subject to a crash. Think of Amazon during the dotcom bubble, where it dropped 90% in market cap value after the bubble burst. Here’s where the third quantitative technique is used, in fulfilling our design goal to avoid the large volatility exhibited by some thematic investing strategies.
To solve this issue, we apply our tried and trusted quantitative alpha model, which we’ve employed successfully over the past 20+ years. With this model, not only are we evaluating each and every company held in our portfolio on the balance of value (reasonably priced), momentum (good price trajectory), quality (the company is profitable), sentiment (the company is viewed positively by the market), and growth (the company is projected to grow in the coming years) metrics, but we do so in a dispassionate manner. Applying the quantitative model allows us to evaluate the investments based on rationality, not hype.
Historical and current detected themes
At any given time, there are many themes playing out in the real world simultaneously. Since the theme detection algorithm we designed is not physically limited like humans, it can detect multiple themes simultaneously and in near real-time.2 Our approach, therefore, invests in multiple themes simultaneously and rotates through themes over time depending on their attractiveness. Figure 2 stylistically illustrates the themes detected by our algorithm, where we see that as time progresses, themes rotate in and out of the detected set. Notice, too, that for themes that exist within the detected set over multiple periods, its width changes from one period to the next. This is because, over time, new companies may enter or exit a given theme, or other themes become more attractive, thus triggering a weight change. For example, the hypothetical internet browser theme was initially composed of Netscape, then later joined by Microsoft and Google as Netscape exited.
Figure 2: Evolution of detected themes
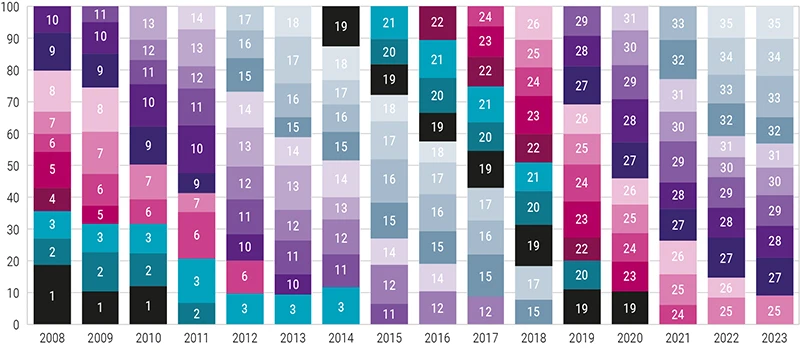
Source: Robeco, 2024.
Figure 3 shows the themes and companies currently detected by our quantitative theme investing process, such as reshaping restaurants, which includes companies like Wingstop, Chipotle and Rational. Their common denominator is their excellence in using digital technologies such as mobile ordering or automated kitchen equipment. This enables them to offer consumers a seamless digital restaurant experience, allowing restaurant operators to be more data-driven and increase profitability.
Figure 3: Current detected themes
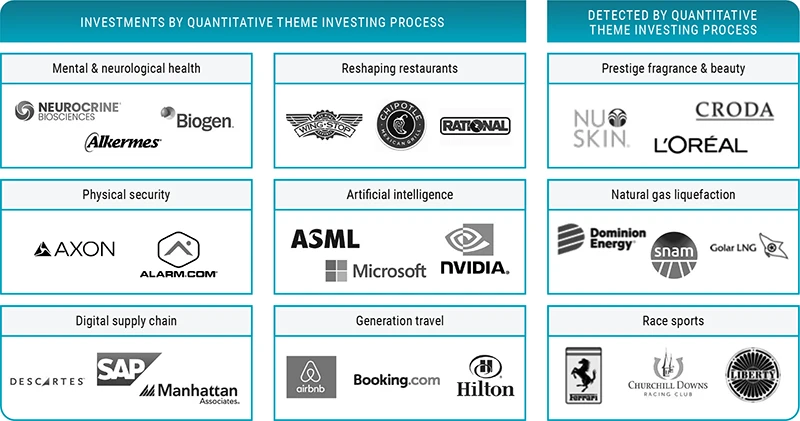
Source: Robeco, 2024.
Despite its sizable advantages, a quantitative approach to thematic investing has certain potential drawbacks compared to a fundamental approach. The biggest drawback is that NLP (and all AI) outputs are probabilistic. This means that while the outputs are likely correct, there are still chances that they could be wrong. In our application, this means both the themes detected and the companies associated with the themes. To address this issue, after the themes and companies are determined algorithmically, experienced portfolio managers oversee the results.
There is a strategic advantage to this combined, ‘quantamental’ process versus simply the fundamental one: it is easier for humans to identify themes and companies that algorithms have detected but do not make sense, than for humans to come up with an extensive set of themes currently occurring in the market place, due to reasons mentioned earlier.
Conclusion
Combining quantitative methods with fundamental PM oversight can enhance the thematic investment process even further. With technology becoming ever more powerful and data ever more readily available, we believe quantitative approaches have much to add.
This article is an excerpt of a special topic in our five-year outlook.
Alle Artikel in dieser Reihe anzeigenFootnotes
1Broadridge. Data per end of Q1 2024.
2The only limits here are the corpora being fed to the algorithm and how well the algorithm is designed in order to detect trending themes.
Webinar: 5-Year Expected Returns 2025-2029
Our five-year outlook on market opportunities and risks.