Choices choices
The paper by Minghui Chen, Matthias Hanauer, and Tobias Kalsbach, titled ‘Design choices, machine learning, and the cross-section of stock returns’, identifies several key design choices researchers have to make when training ML models. For instance, when setting the prediction (target) variable, should the researcher employ the excess return over the risk-free rate or the abnormal return relative to the market? Is it better to use a continuous target variable or are categories, such as outperformers vs. underperformers, preferable? Is it better to train models based on a rolling window that leads to more adaptive models, or are models based on expanding windows superior, thanks to the availability of more training data?
To assess the importance of such choices, the authors identify seven such key design choices and examine all the ensuing possible combinations, resulting in a total of 1,056 ML models. In this way, the study trains each model on a common set of signals (features) for the US stock market and evaluates their out-of-sample performance using hypothetical top-minus-bottom decile portfolios.
Figure 1 reveals that portfolio returns vary substantially across different model designs, with monthly mean returns ranging from 0.13% to 1.98% and annualized Sharpe ratios ranging from 0.08 to 1.82.1 This variation highlights the substantial impact of human design choices on the performance of ML strategies.
Figure 1 | Cumulative performance of machine learning strategies
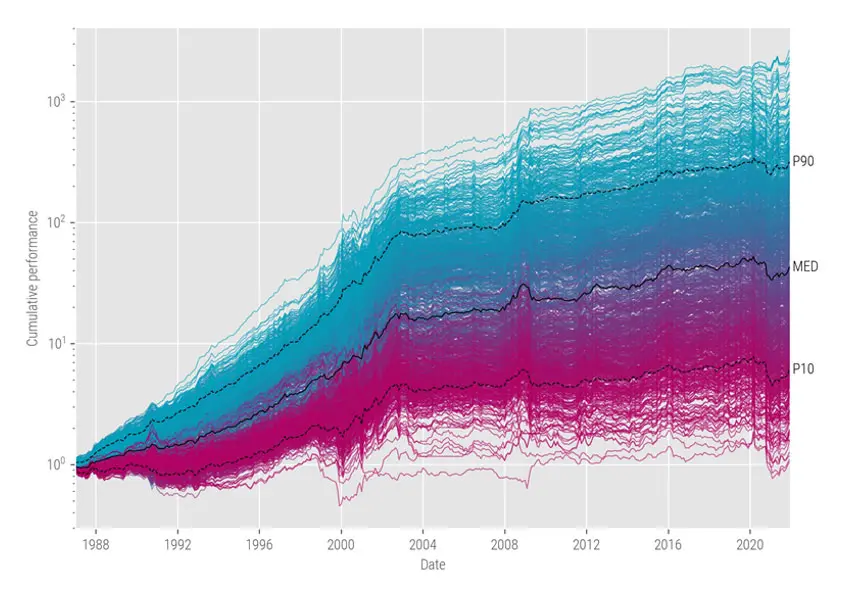
Source: Robeco, Chen et al. (2024). This figure shows the cumulative performance of a USD 1 initial investment in long-short ML portfolios for each possible combination of the research design choices. For each ML model and month, we first cross-sectionally sort all stocks based on their one-month-ahead return predictions. We then construct the value-weighted long-short portfolios by going long the top decile and short the bottom decile stocks. The solid black line represents the strategy with the median cumulative performance for each month, and the dashed black lines represent the 10th and 90th percentiles of each month, respectively. The sample period is from January 1987 to December 2021.
Machine learning models: Separating the wheat from the chaff
Having documented the substantial variation in the performance of ML models, the study also provides actionable guidance for ML model design:
Ensembles of ML models typically outperform individual algorithms.
The choice of target variable depends on the investment objective:
o For identifying relative winners and losers among stocks, predicting stock returns over the market rather than the risk-free rate is better.
o If the goal is to achieve high market-risk-adjusted returns, CAPM beta-adjusted returns are better.Non-linear ML models are more likely to outperform their linear counterparts when:
o using abnormal returns relative to the market as the target variable,
o employing continuous target returns, or
o adopting expanding training windows.
Conclusion
While computational infrastructure, ML algorithms, and data have become significantly more accessible over the past decade or two, model design remains a critical component of success. At first glance, it might seem that an ML investment strategy only requires a few basic elements: cloud computing space, generic factor data, some Python packages, and a couple of data scientists. However, this approach often lacks the crucial domain knowledge that Robeco has cultivated over 20 years in quant investing. That’s why in financial markets, where the signal-to-noise ratio is low and the risk of overfitting high, investment experience, and economic intuition still play a pivotal role. Robeco’s extensive expertise ensures that ML models focus on meaningful patterns and avoid common pitfalls, bridging the gap between technology and investment insight.
Footnote
1Please note that these are hypothetical gross returns for long-minus-short strategies that do not consider any transaction costs. We investigated the impact of transaction costs on ML strategies in our study ‘The term structure of machine learning alpha’.
クオンツ運用の価値を探求
最先端クオンツ戦略の情報やインサイトを定期的にお届けします。
重要事項
当資料は情報提供を目的として、Robeco Institutional Asset Management B.V.が作成した英文資料、もしくはその英文資料をロベコ・ジャパン株式会社が翻訳したものです。資料中の個別の金融商品の売買の勧誘や推奨等を目的とするものではありません。記載された情報は十分信頼できるものであると考えておりますが、その正確性、完全性を保証するものではありません。意見や見通しはあくまで作成日における弊社の判断に基づくものであり、今後予告なしに変更されることがあります。運用状況、市場動向、意見等は、過去の一時点あるいは過去の一定期間についてのものであり、過去の実績は将来の運用成果を保証または示唆するものではありません。また、記載された投資方針・戦略等は全ての投資家の皆様に適合するとは限りません。当資料は法律、税務、会計面での助言の提供を意図するものではありません。 ご契約に際しては、必要に応じ専門家にご相談の上、最終的なご判断はお客様ご自身でなさるようお願い致します。 運用を行う資産の評価額は、組入有価証券等の価格、金融市場の相場や金利等の変動、及び組入有価証券の発行体の財務状況による信用力等の影響を受けて変動します。また、外貨建資産に投資する場合は為替変動の影響も受けます。運用によって生じた損益は、全て投資家の皆様に帰属します。したがって投資元本や一定の運用成果が保証されているものではなく、投資元本を上回る損失を被ることがあります。弊社が行う金融商品取引業に係る手数料または報酬は、締結される契約の種類や契約資産額により異なるため、当資料において記載せず別途ご提示させて頂く場合があります。具体的な手数料または報酬の金額・計算方法につきましては弊社担当者へお問合せください。 当資料及び記載されている情報、商品に関する権利は弊社に帰属します。したがって、弊社の書面による同意なくしてその全部もしくは一部を複製またはその他の方法で配布することはご遠慮ください。 商号等: ロベコ・ジャパン株式会社 金融商品取引業者 関東財務局長(金商)第2780号 加入協会: 一般社団法人 日本投資顧問業協会