In the past five years, the application of machine learning (ML) techniques for predicting stock returns has seen a significant surge. Numerous studies have confirmed that ML-based alpha models often outperform traditional, linear models in predicting cross-sectional equity returns.1 However, ML techniques are often referred to as “black boxes.” Something goes in, something comes out, but the inner workings of the algorithms remain obscure. This is where tools like Shapley values come into play – they help to understand why machine-learning models make certain predictions.2
For every prediction an ML model makes, Shapley values indicate the contribution of each variable (feature) to the prediction (target). Imagine we’re predicting future stock returns, and the model predicts an outperformance of 4% for a particular stock. Shapley values allow us to attribute this for instance as follows: 2% due to value, 1% due to momentum, and 1% due to quality.
Figure 1: Shapley plots for a boosted regression tree model predicting one-month-ahead returns.
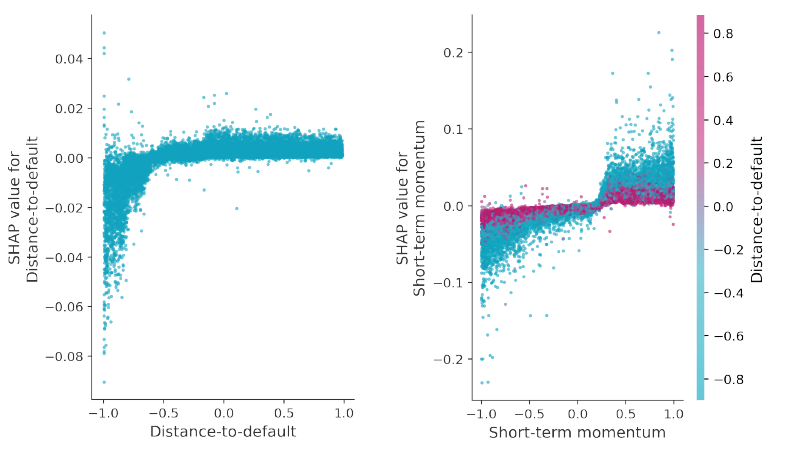
Source: Robeco, Refinitiv. The figure shows a Shapley scatter plot (left) and a Shapley dependence plot (right) for a boosted regression tree model predicting one-month ahead standardized returns. Shapley values are shown on the y-axis, with a Shapley value above 0 indicating that a feature has a positive impact on model predictions. The chart on the left shows the relation between distance-to-default and one-month-ahead returns. The chart on the right shows an interaction effect between short-term momentum (x-axis) and distance-to-default (color) for one-month-ahead returns. The boosted regression tree model is trained on one-month ahead relative returns. We include several dozen common as well as proprietary features whose ranks are cross-sectionally mapped into the [-1,1] interval. For missing values, the cross-sectional median is imputed. The model is trained on monthly data from January 1986 to December 2022, using all constituents of the MSCI World Index.
As technology advances, so do the opportunities for quantitative investors. By incorporating more data and leveraging advanced modelling techniques, we can develop deeper insights and enhance decision-making.
Furthermore, Shapley dependence plots can also illuminate the functional form between a feature and the target. Figure 1, for instance, illustrates potential nonlinearities and interaction effects in ML return prediction models. Shapley values are shown on the y-axis, with a Shapley value above 0 indicating that a feature has a positive impact on model predictions.
The chart on the left indicates a positive relationship between distance-to-default and expected returns. This pattern is consistent with the well-known low-risk effect, which suggests that higher risks are not necessarily rewarded with higher returns. However, this relationship is nonlinear: stocks closer to default exhibit a highly negative relation between distress risk and expected returns, while the relationship remains relatively flat for stocks far away from default.
Moreover, the chart on the right unveils an interaction effect between short-term momentum and distance-to-default.3 Generally, the Shapley plot indicates that stocks with high short-term momentum tend to have higher future returns. However, this effect is more pronounced for stocks with low distance-to-default (blue dots) than for stocks with high distance-to-default (red dots). This insight reveals that while stocks with low distance-to-default typically have lower expected returns, short-term momentum can discern between short-term winners and losers within this volatile group of stocks.
In conclusion, Shapley values play a pivotal role in transforming ML models from “black boxes” to “glass boxes.” The black boxes metaphor stems from the increased complexity of ML models and the difficulty in understanding the decision-making process behind predictions. Shapley values, however, quantify the contribution of each feature in the model to a specific prediction. They provide a transparent layer, allowing us to see and understand the impact and importance of individual variables on the predictions. This interpretability, akin to peering into a glass box, is paramount in assessing the trustworthiness of ML predictions and making informed investment decisions based on them.
Footnotes
1 See for instance, Gu, Kelly, and Xiu, 2020, “Empirical Asset Pricing via Machine Learning”, The Review of Financial Studies for the United States, Tobek and Hronec, 2021, “Does it pay to follow anomalies research? Machine learning approach with international evidence”, Journal of Financial Markets for developed markets, and Hanauer and Kalsbach, 2023, “Machine learning and the cross-section of emerging market stock returns”, Emerging Markets Review for emerging markets. For a discussion of the promises and pitfalls of ML, we also refer to and Leung, Lohre, Mischlich, Shea, and Stroh, 2021, “The Promises and Pitfalls of Machine Learning for Predicting Stock Returns”, The Journal of Financial Data Science, Blitz, Hoogteijling, and Lohre, 2023, “Researchers have just been scratching the surface of ML in asset management”, Robeco article, and Chen and Zhou, 2023, “Machine learning in finance: Why and how?”, Robeco article.
2 See Shapley, 1953. “A Value for n-person Games.” Contributions to the Theory of Games. Annals of Mathematical Studies.
3 Short-term momentum is a proprietary signal with a lookback of one month that captures systematic short-term momentum effects such as industry, country, and factor momentum.
Quant Charts
重要事項
当資料は情報提供を目的として、Robeco Institutional Asset Management B.V.が作成した英文資料、もしくはその英文資料をロベコ・ジャパン株式会社が翻訳したものです。資料中の個別の金融商品の売買の勧誘や推奨等を目的とするものではありません。記載された情報は十分信頼できるものであると考えておりますが、その正確性、完全性を保証するものではありません。意見や見通しはあくまで作成日における弊社の判断に基づくものであり、今後予告なしに変更されることがあります。運用状況、市場動向、意見等は、過去の一時点あるいは過去の一定期間についてのものであり、過去の実績は将来の運用成果を保証または示唆するものではありません。また、記載された投資方針・戦略等は全ての投資家の皆様に適合するとは限りません。当資料は法律、税務、会計面での助言の提供を意図するものではありません。 ご契約に際しては、必要に応じ専門家にご相談の上、最終的なご判断はお客様ご自身でなさるようお願い致します。 運用を行う資産の評価額は、組入有価証券等の価格、金融市場の相場や金利等の変動、及び組入有価証券の発行体の財務状況による信用力等の影響を受けて変動します。また、外貨建資産に投資する場合は為替変動の影響も受けます。運用によって生じた損益は、全て投資家の皆様に帰属します。したがって投資元本や一定の運用成果が保証されているものではなく、投資元本を上回る損失を被ることがあります。弊社が行う金融商品取引業に係る手数料または報酬は、締結される契約の種類や契約資産額により異なるため、当資料において記載せず別途ご提示させて頂く場合があります。具体的な手数料または報酬の金額・計算方法につきましては弊社担当者へお問合せください。 当資料及び記載されている情報、商品に関する権利は弊社に帰属します。したがって、弊社の書面による同意なくしてその全部もしくは一部を複製またはその他の方法で配布することはご遠慮ください。 商号等: ロベコ・ジャパン株式会社 金融商品取引業者 関東財務局長(金商)第2780号 加入協会: 一般社団法人 日本投資顧問業協会